
Using machine learning to speed up discovery for drug delivery and disease treatment
A new computational tool developed with support from the U.S. National Science Foundation could greatly speed up determining the 3D structure of RNAs, a critical step in developing new RNA-based drugs, identifying drug-binding sites and using RNAs in other biotechnology and biomedicine applications.
The tool, NuFold, leverages state-of-the-art machine learning techniques to predict the structure of a wide variety of RNA molecules from their sequences. This new capability will allow researchers to visualize what a given RNA structure could look like based on its sequence and identify its potential use in drug delivery, disease treatment and other applications. The research leading to NuFold was published in Nature Communications.
RNAs are critical biological molecules — encoding information, like DNA, and performing cellular functions, like proteins — but relatively few RNA structures have been determined through experimentation thus far, which severely limits understanding of their functions. For example, RNAs in the NSF-funded Research Collaboratory for Structural Bioinformatics Protein Data Bank (RCSB PDB) represent only about 3% of total entries. Experimentally determining RNA structures is often time-consuming and costly. By providing a path to reliably predicting RNA structure from sequence, NuFold could greatly expedite the discovery of RNA function and enable quicker development of RNA-based therapeutics and technologies.
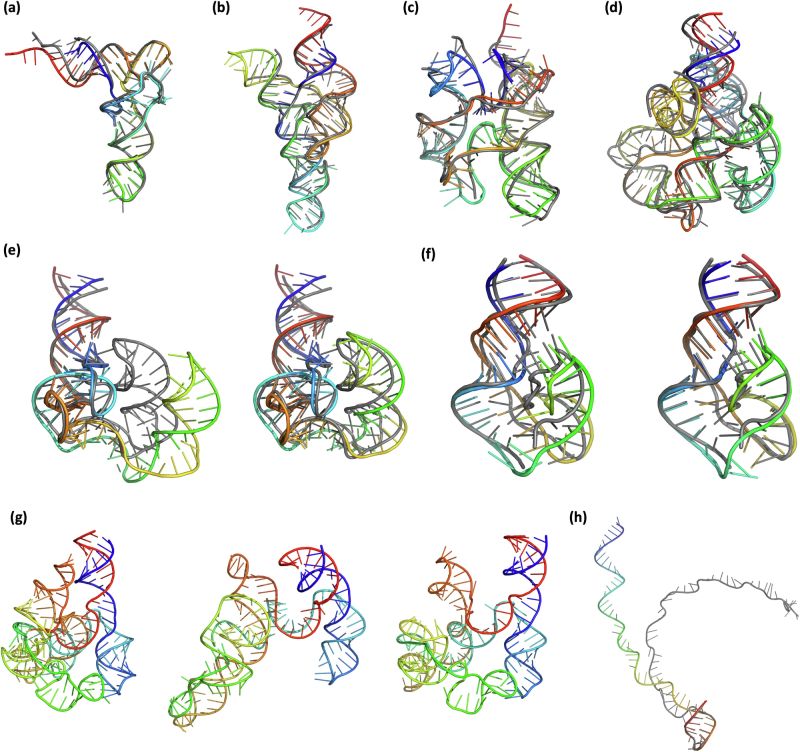
Credit: Daisuke Kihara, Purdue University. Figure taken from the Nufold paper under a Creative Commons Attribution-NonCommercial-NoDerivatives 4.0 International License
These therapeutics and technologies could help address a range of diseases and conditions. For example, information on the structure of small interfering RNAs could aid in limiting gene overexpression that can play a role in cancer, neurological disorders and kidney stones. Knowing the structure of RNAs also could help enhance food security by protecting plants from viruses.
NuFold leverages state-of-the-art machine learning techniques to predict the structure of a range of RNA molecules from their sequences. The system architecture for NuFold is based on the artificial intelligence-based protein structure prediction tool AlphaFold2, which was trained on the RCSB PDB and whose developers were awarded the 2024 Nobel Prize in chemistry. The source code for NuFold is openly available for use by the broad computational biology research community and other researchers interested in RNA structures.
The machine-learning-enabled 3D RNA structures can be realized through novel 3D nanomanufacturing approaches.
Learn more about NSF support for biotechnology.

Distribution channels: Science
Legal Disclaimer:
EIN Presswire provides this news content "as is" without warranty of any kind. We do not accept any responsibility or liability for the accuracy, content, images, videos, licenses, completeness, legality, or reliability of the information contained in this article. If you have any complaints or copyright issues related to this article, kindly contact the author above.
Submit your press release